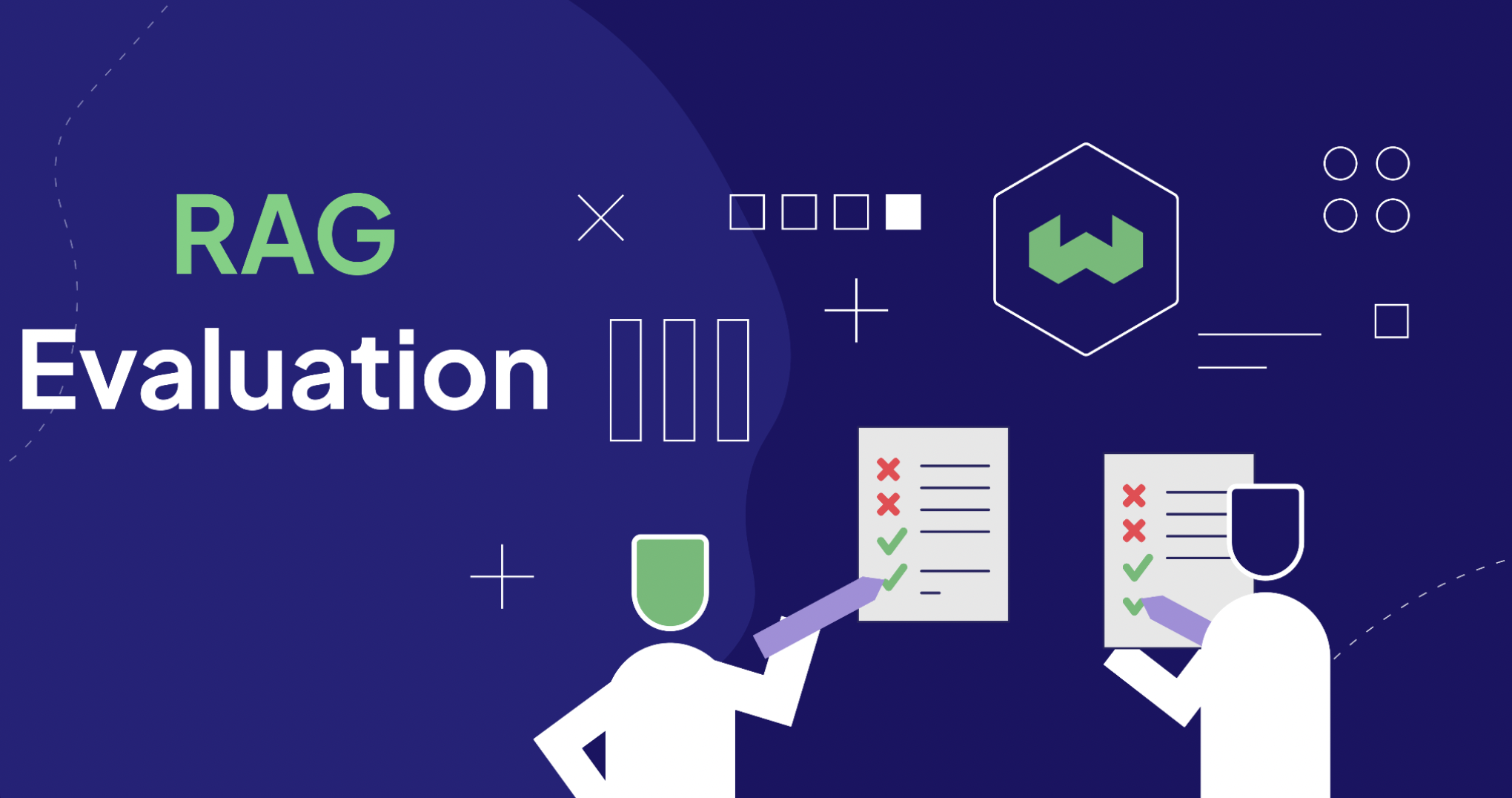
Meta has introduced an innovative factual question-answering (QA) benchmark that promises to enhance the evaluation of Retrieval-Augmented Generation (RAG) systems used in large language models (LLMs). This benchmark encompasses a comprehensive set of 4,409 diverse questions, providing a robust framework for assessing the accuracy and effectiveness of QA systems.
Understanding RAG Systems
Retrieval-Augmented Generation systems represent a hybrid approach to question answering, combining the strengths of both retrieval-based and generation-based models. In this framework, a retrieval component first gathers relevant documents or pieces of information from a large corpus. Subsequently, a generation component uses this retrieved information to construct accurate and contextually appropriate answers. This approach aims to leverage the precision of retrieval models with the flexibility and fluency of generative models.
The Need for a Comprehensive Benchmark
The complexity and diversity of questions posed to QA systems have grown significantly, necessitating more rigorous and realistic evaluation benchmarks. Meta’s new benchmark addresses this need by offering a diverse array of questions, each designed to test different aspects of a system’s capabilities. These questions span various domains and formats, ensuring a well-rounded assessment of the system’s performance.
Features of Meta’s QA Benchmark
- Diverse Question Set: The 4,409 questions included in the benchmark are meticulously curated to cover a broad spectrum of topics. This diversity ensures that the evaluation is not biased towards any specific domain, providing a more accurate measure of a system’s general capabilities.
- Mock APIs: To simulate real-world scenarios, Meta’s benchmark includes mock APIs. These APIs are designed to mimic the behavior of actual information sources, challenging the QA systems to retrieve and synthesize information in a realistic manner. This feature is crucial for testing how well systems can handle dynamic and varied data sources.
- Realistic Challenges: The benchmark is designed to present realistic challenges that a QA system might encounter in practical applications. These challenges include ambiguous questions, incomplete information, and the need to integrate data from multiple sources. By tackling these issues, the benchmark ensures that the evaluated systems are robust and versatile.
Implications for LLM Development
Meta’s factual QA benchmark is poised to play a significant role in the development and refinement of LLMs. By providing a standardized and challenging evaluation framework, it enables researchers and developers to identify strengths and weaknesses in their systems. This, in turn, fosters the creation of more reliable and efficient QA systems capable of performing in real-world conditions.
Conclusion
The introduction of Meta’s factual QA benchmark marks a significant advancement in the field of natural language processing. By offering a diverse set of questions, realistic mock APIs, and challenging scenarios, the benchmark provides a comprehensive tool for evaluating the performance of RAG systems in LLMs. As a result, it is expected to drive significant improvements in the development of QA systems, ultimately leading to more accurate and reliable AI-powered solutions in various domains.